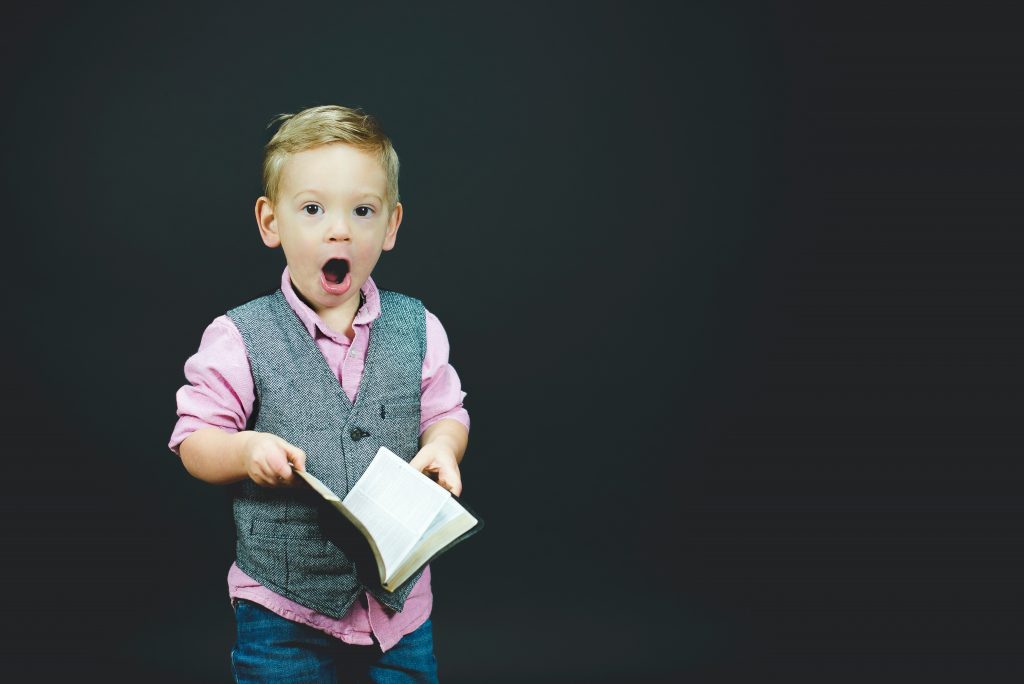
We will eventually invent a practical, driverless car. People talk about the repercussions of that invention. It will make life more convenient. It will reduce vehicular deaths. It might reduce the number of people owning cars. It might reduce the vehicles on the road and the carbon footprint of cars. It will reduce the need for people whose job it is to drive. We have the technology and knowledge to make this giant technological leap, and we’re investing billions because the rewards are enormous.
We have the opportunity to bring the population along for the ride or leave them at the curbside. The same technologies used to make driverless cars safe and useful can help educate society. This idea may not be obvious, so let me help you make the leap.
Learning From Doing
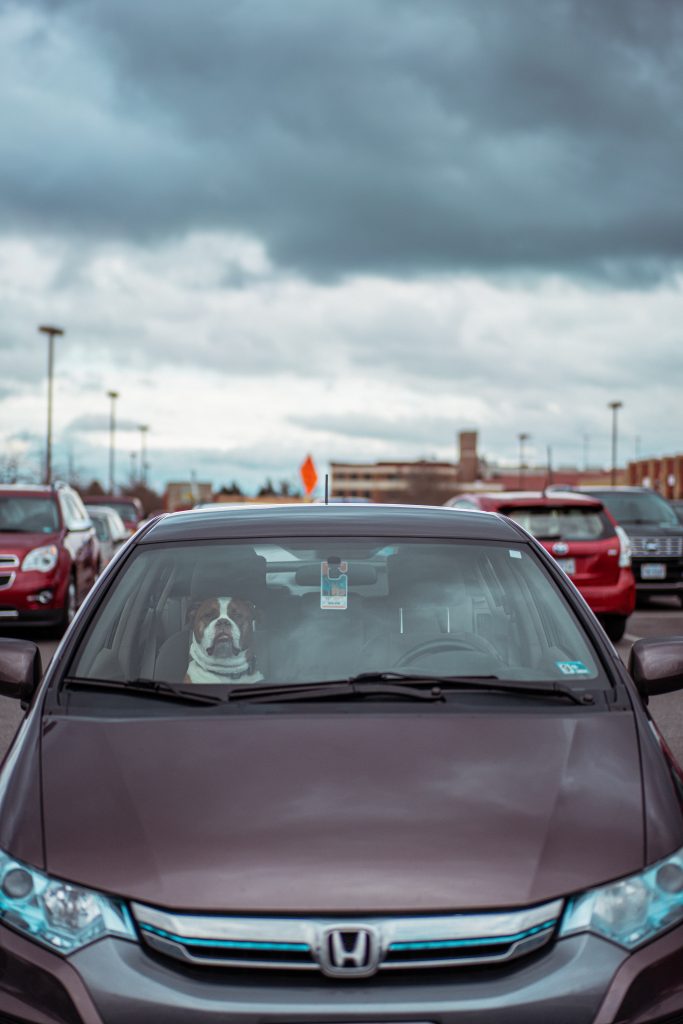
Driverless cars, as well as many kinds of automation, use a type of artificial intelligence that you have no doubt heard called machine learning. An oversimplified example of how this works looks like this:
- Drive a car around town and collect data from sensors about what the vehicle encounters along its route.
- Process the data to create a model of how the vehicle should behave the next time it drives around town.
- Drive the car around in new scenarios while assisted by the new model and collect more data.
- Create a feedback loop by redoing steps 2 and 3 repeatedly until the car can drive itself without any problems.
Each time the model gets better with more scenarios and more data. The car “learns” how to stop at stop signs, avoid pedestrians, and even how to react in construction sites where people are directing with flags. To prevent physical accidents, companies are turning to video game technologies to recreate the driving scenarios inside computers to generate more data faster.
Data Collection for Educational Assessment
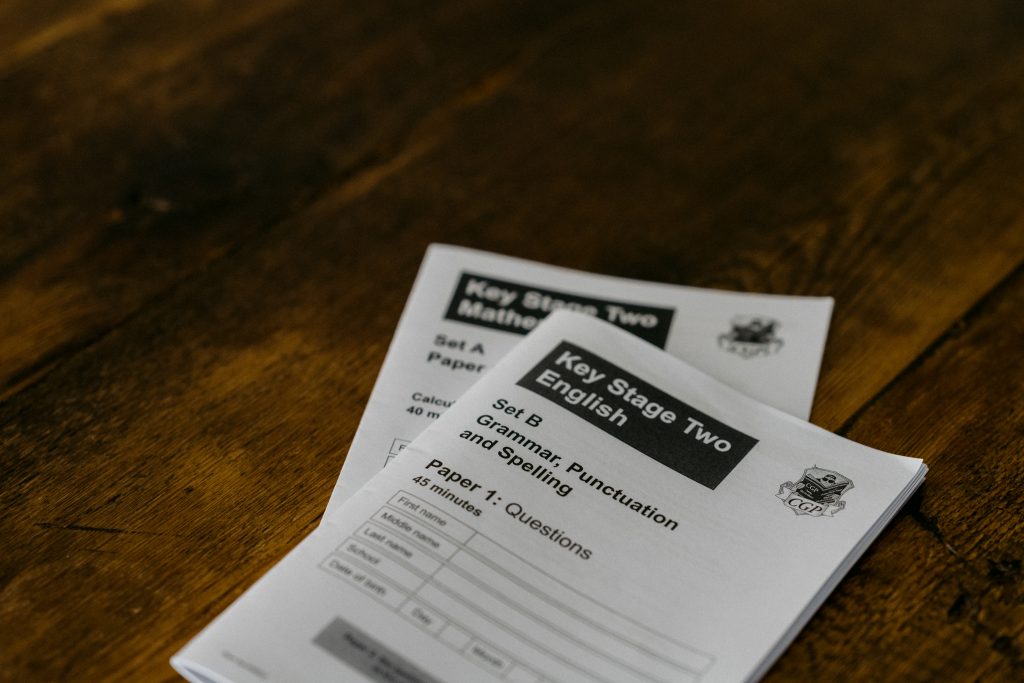
Now let’s apply the same technology to learning. The first thing we need to do is to collect data about any teaching that happens and learn from the outcomes. How do we collect data now? Through assessments and testing. Assessments generally occur in the classroom or a counselor’s office. They happen in controlled environments, in group observation or one-on-one, and take time, effort, and training. You can probably already guess that the accuracy of the data collected from these assessments is sometimes problematic. The regularity and reliability can vary significantly between assessments based on many factors, including the number of kids in the classroom and the training/skills of the assessors.
Armed with real-time assessment data, teachers, administrators, and families can implement interventions and launch new, more successful educational journeys that will help the student succeed now.
What about testing? Standardized testing generally happens at regular intervals throughout the year, and data is collected. Most schools test for mastery according to pre-set standards and growth of the student’s knowledge. Suppose, for this discussion, that we assume the tests are unbiased – something we recognize is in dispute. Many of the standardized tests are not, generally speaking, for the student’s benefit but rather intended so that the institution can measure its progress. This strategy has the opportunity to help future generations of students, but not the student who just sat through the test. The difficulty comes when trying to link the results to useful feedback for the student; generally, the test results arrive after the classroom has moved on from the subject, leaving no opportunity to work on the feedback provided. For some students, standardized tests are like placing a detour sign 10 miles after the turnoff.
Wealth Disparities in Data Collection and Interventions
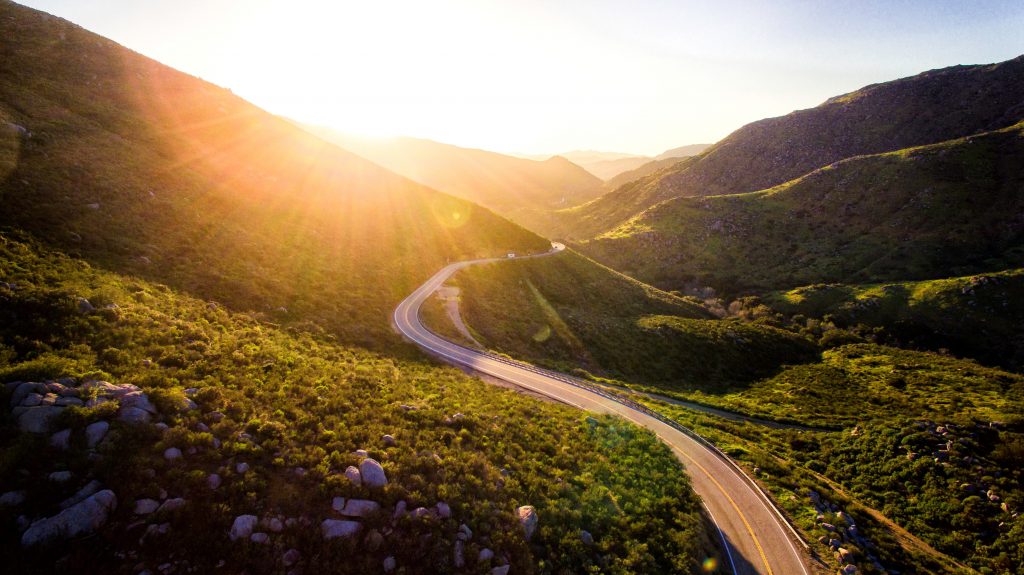
If we go back to the automated driverless car, the models improve with the faster and more robust collection of reliable data. We can probably assume that the more reliable the information parents and teachers have about a child, the better the interventions and the better the outcomes. Some teachers in wealthier communities have more time to spend assessing each child. More affluent parents can generally spend more time helping, assisting the student, and can afford outside assistance. Thus, more data is readily available in higher-income areas. The evidence of these disparities shows up in the extremely high correlation between wealth and test scores. The information itself is not biased towards the rich; the access and collection are biased towards the rich.
No Data, No Models, No Improvement
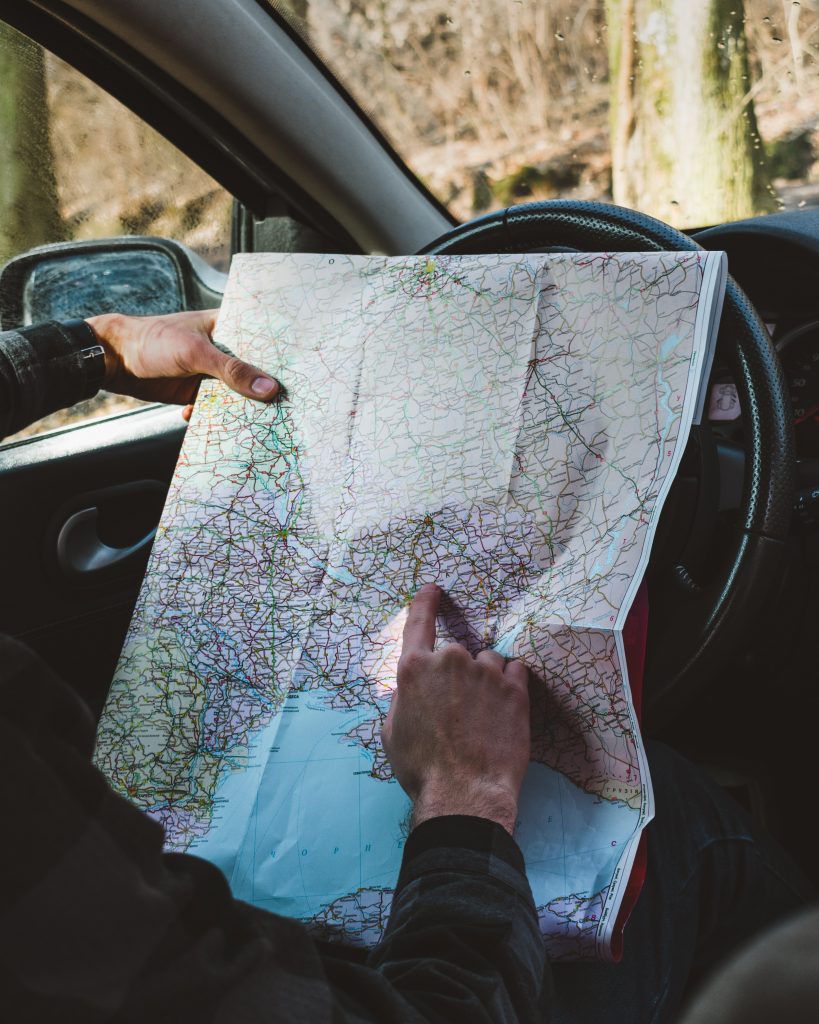
Without regular, reliable, and timely data, how can we create the feedback loop to help? We need to start by changing the ways and frequency of collecting the information needed for our models. Learning that your child is not meeting peer standards at the quarterly parent-teacher conference is a concept so antiquated it is doing a vast disservice to our communities. As a comparable example, Waze can tell me in moments whether the traffic jam ahead is going to cause me a 5-minute delay. Waze knows because it collects data from thousands of other travelers, feeds that data into its ever-improving model, and informs me of the issues. There are 76 million students in the United States alone. Surely we can collect data faster and provide feedback in real-time for people to navigate a better path on their educational journey.
The consequences are real. We will create a fleet of autonomous cars in which no one can afford to ride. While society evolves to use technology to automate away jobs that involve repetitive tasks, we need to flexibly educate the population to use their brains in new and as-yet-undetermined ways. But for some people without access to data or knowledge, they’re like exit ramps off the education highway without a map.
Examining and acting on assessment data is only the tip of the iceberg for securing student success. Ask any teacher why their students don’t always live up to their expectations, and they will likely identify a myriad of social-emotional, community, and societal factors. There’s data there too we can use, and we’ll talk more about the data of student engagement in the next installment!
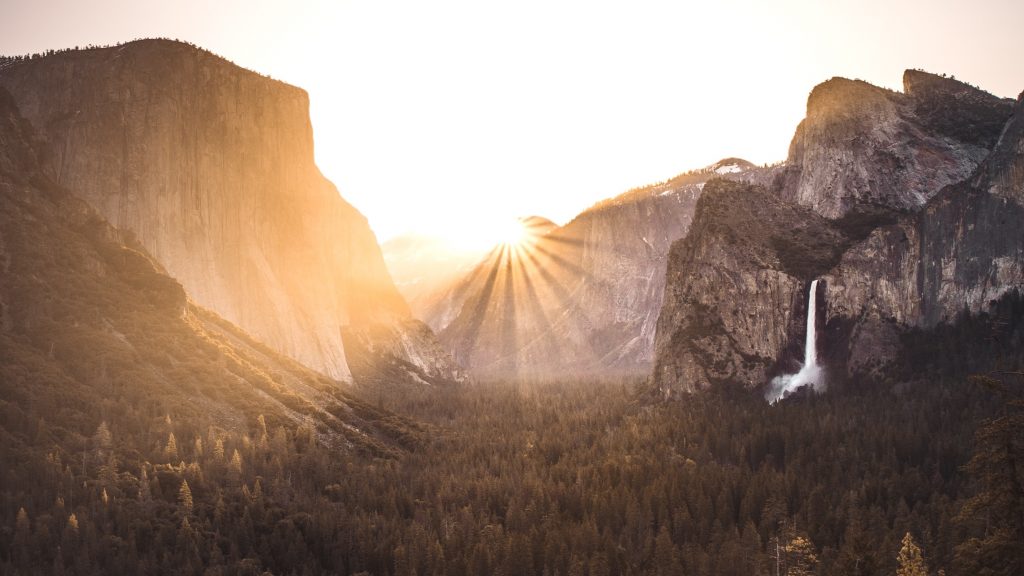